A few weeks ago, we discussed one of AIXPRT’s key configuration variables, batch size. Today, we’re discussing another key variable: the level of precision. In the context of machine learning (ML) inference, the level of precision refers to the computer number format (FP32, FP16, or INT8) representing the weights (parameters) a network model uses when performing the calculations necessary for inference tasks.
Higher levels of precision for inference tasks help decrease the number of false positives and false negatives, but they can increase the amount of time, memory bandwidth, and computational power necessary to achieve accurate results. Lower levels of precision typically (but not always) enable the model to process inputs more quickly while using less memory and processing power, but they can allow a degree of inaccuracy that is unacceptable for certain real-world applications.
For example, a high level of precision may be appropriate for computer vision applications in the medical field, where the benefits of hyper-accurate object detection and classification far outweigh the benefit of saving a few milliseconds. On the other hand, a low level of precision may work well for vision-based sensors in the security industry, where alert time is critical and monitors simply need to know if an animal or a human triggered a motion-activated camera.
FP32, FP16, and INT8
In AIXPRT, we can instruct the network models to use FP32, FP16, or INT8 levels of precision:
- FP32 refers to single-precision (32-bit) floating point format, a number format that can represent an enormous range of values with a high degree of mathematical precision. Most CPUs and GPUs handle 32-bit floating point operations very efficiently, and many programs that use neural networks, including AIXPRT, use FP32 precision by default.
- FP16 refers to half-precision (16-bit) floating point format, a number format that uses half the number of bits as FP32 to represent a model’s parameters. FP16 is a lower level of precision than FP32, but it still provides a great enough numerical range to successfully perform many inference tasks. FP16 often requires less time than FP32, and uses less memory.
- INT8 refers to the 8-bit integer data type. INT8 data is better suited for certain types of calculations than floating point data, but it has a relatively small numeric range compared to FP16 or FP32. Depending on the model, INT8 precision can significantly improve latency and throughput, but there may be a loss of accuracy. INT8 precision does not always trade accuracy for speed, however. Researchers have shown that a process called quantization (i.e., approximating continuous values with discrete counterparts) can enable some networks, such as ResNet-50, to run INT8 precision without any significant loss of accuracy.
Configuring precision in AIXPRT
The screenshot below shows part of a sample config file, the same sample file we used for our batch size discussion. The value in the “precision” row indicates the precision setting. This test configuration would run tests using INT8. To change the precision, a tester simply replaces that value with “fp32” or “fp16” and saves the changes.
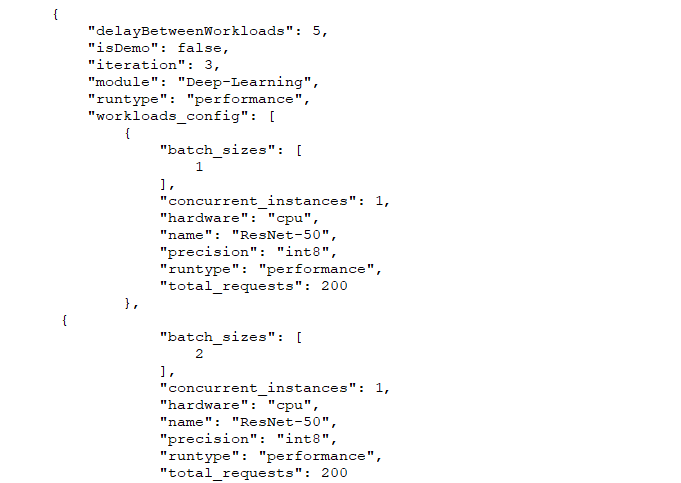
Note that while decreasing the precision from FP32 to FP16 or INT8 often results in larger throughput numbers and faster inference speeds overall, this is not always the case. Many other factors can affect ML performance, including (but not limited to) the complexity of the model, the presence of specific ML optimizations for the hardware under test, and any inherent limitations of the target CPU or GPU.
As with most AI-related topics, the details of model precision are extremely complex, and it’s a hot topic in cutting edge AI research. You don’t have to be an expert, however, to understand how changing the level of precision can affect AIXPRT test results. We hope that today’s discussion helped to make the basics of precision a little clearer. If you have any questions or comments, please feel free to contact us.
Justin