Last week, we discussed the changes we made to the AIXPRT Community Preview 2 (CP2) download page as part of our ongoing effort to make AIXPRT easier to use. This week, we want to discuss the basics of understanding AIXPRT results by talking about the numbers that really matter and how to access and read the actual results files.
To understand AIXPRT results at a high level, it’s important to revisit the core purpose of the benchmark. AIXPRT’s bundled toolkits measure inference latency (the speed of image processing) and throughput (the number of images processed in a given time period) for image recognition (ResNet-50) and object detection (SSD-MobileNet v1) tasks. Testers have the option of adjusting variables such as batch size (the number of input samples to process simultaneously) to try and achieve higher levels of throughput, but higher throughput can come at the expense of increased latency per task. In real-time or near real-time use cases such as performing image recognition on individual photos being captured by a camera, lower latency is important because it improves the user experience. In other cases, such as performing image recognition on a large library of photos, achieving higher throughput might be preferable; designating larger batch sizes or running concurrent instances might allow the overall workload to complete more quickly.
The dynamics of these performance tradeoffs ensure that there is no single good score for all machine learning scenarios. Some testers might prefer lower latency, while others would sacrifice latency to achieve the higher level of throughput that their use case demands.
Testers can find latency and throughput numbers for each completed run in a JSON results file in the AIXPRT/Results folder. The test also generates CSV results files that are in the same folder. The raw results files report values for each AI task configuration (e.g., ResNet-50, Batch1, on CPU). Parsing and consolidating the raw data can take some time, so we’re developing a results file parsing tool to make the job much easier.
The results parsing tool is currently available in the AIXPRT CP2 OpenVINO – Windows package, and we hope to make it available for more packages soon. Using the tool is as simple as running a single command, and detailed instructions for how to do so are in the AIXPRT OpenVINO on Windows user guide. The tool produces a summary (example below) that makes it easier to quickly identify relevant comparison points such as maximum throughput and minimum latency.

In addition to the summary, the tool displays the throughput and latency results for each AI task configuration tested by the benchmark. AIXPRT runs each AI task multiple times and reports the average inference throughput and corresponding latency percentiles.
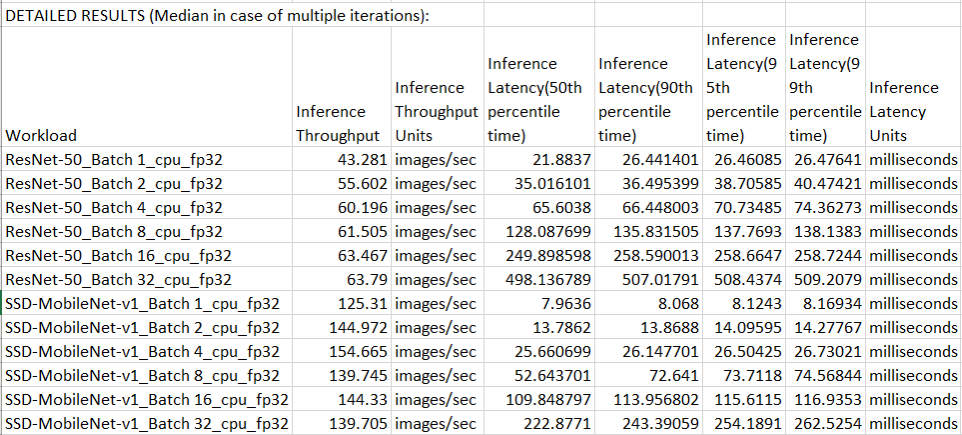
We hope that this information helps to make it easier to understand AIXPRT results. If you have any questions or comments, please feel free to contact us.
Justin